In the winter of 1997 Carver Mead lectured on an unusual topic for a computer scientist: the nervous systems of animals, such as the humble fly. Mead, a researcher at the California Institute of Technology, described his earlier idea for an electronic problem-solving system inspired by nerve cells, a technique he had dubbed “neuromorphic” computing. A quarter-century later, researchers have designed a carbon-based neuromorphic computing device—essentially an organic robot brain—that can learn to navigate a maze.
A neuromorphic chip memorizes information similarly to the way an animal does. When a brain learns something new, a group of its neurons rearrange their connections so they can communicate more quickly and easily. As a common saying in neuroscience goes, “Neurons that fire together wire together.” When a neuromorphic chip learns, it rewires its electric circuits to save the new behavior like a brain does to save a memory.
The idea of brainlike computation has been around for a while. But Paschalis Gkoupidenis of the Max Planck Institute for Polymer Research in Mainz, Germany, and his neuromorphic research team are pioneers in crafting this technology from organic materials. To build their chip, the researchers used long chains of carbon-based molecules called polymers, which are soft and, in some ways, behave similarly to living tissues. In order to let their material carry an electric charge like real neurons, which are energy-efficient and operate in a watery medium, the scientists coated the organic material with an ion-rich gel. This provided “more degrees of freedom to mimic biological processes,” Gkoupidenis says.
Previously, some of the researchers who worked with Gkoupidenis’s Max Planck group on the new study had shown that organic polymers can record aspects of their past states. This finding had suggested that the polymers can “remember” certain information, such as the sequence of turns required to navigate a maze. So in the recent investigation, the team used organic material to construct transistors—power- and signal-switching devices—and arranged them into a circuit. The resulting “brain chip” can receive sensory signals and use them to adapt to environmental stimuli. After it has learned which way to move, the circuit can send precise motor commands to a robot body. The researchers described their work in Science Advances last month.
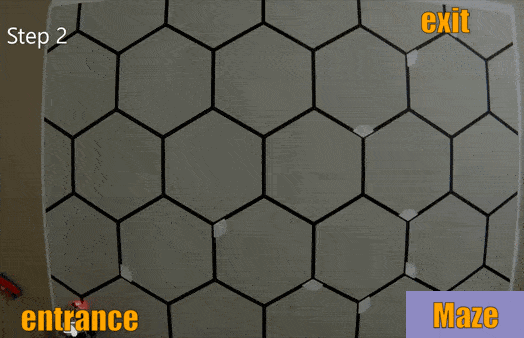
Once the team members had designed their organic robot brain chip, a maze seemed like the perfect real-world situation in which to test it. This is because success or failure becomes obvious immediately: if the robot finishes the maze, it has clearly learned something—and “if it doesn’t, then it didn’t learn,” explains study co-author Yoeri van de Burgt of Eindhoven University of Technology in the Netherlands.
The team selected a commercial toy robot called Lego Mindstorms EV3, which has two input sensors to register signals for touch and “sight” and two wheels to move around. The scientists equipped the toy with their chip, which could control the direction in which the wheels moved. Then they designed a two-square-meter maze that looked like a two-dimensional honeycomb, filled with potential crossroads, and turned the robot loose in it.
At each crossroad, the machine turned right by default. But each time it eventually hit a side wall, it received a “slap on the nose,” as van de Burgt puts it. “Well, that’s a fancy [phrase] for basically tuning the resistance a little bit,” he adds. This means that when the robot was given a light human tap or hit a wall, the sensors carried that touch signal to the organic circuit. In response—like neurons rewiring after they receive a corrective stimulus—an electric property of the polymer called resistance was reduced. This allowed more voltage to pass through the polymer, which energized the ions in the material to move to another end of the circuit. Based on the movement and accumulation of ions, the robot brain could now make a different decision: at the intersection that originally tripped it up, instead of turning right by default, it would now turn left. In this way, the robot learned. With each wrong move, the robot either hit a wall or was gently touched by the researchers. Then it was moved back to the start of the maze. The robot kept learning which way to turn at each new crossing until, at the end of the 16th run, it finally made it to the exit.
“The device learns in the same way we teach kids, giving rewards if they are correct or not rewarding if they are wrong,” says Arindam Basu, a professor of electrical engineering at the City University of Hong Kong, who was not involved in the new study. In this case, the robot only performed binary decisions, turning either left or right. “So it would be interesting to extend the task to choose between multiple decisions,” Basu says.
The experiment is “really cool,” says Jeffrey Krichmar, a computer scientist at the University of California, Irvine, who was also not involved in the study. The robot was allowed to make mistakes and amend them later on, Krichmar says. The researchers did not preprogram its future steps, he notes, “but they let the whole training be a part of its circuit.”
Although the experiment demonstrated the learning power of an organic control chip, the machine’s ability to sense its surroundings and move still relied on the inorganic components of the toy robot. “Next steps could be replacing them with organic counterparts,” says Robert Nawrocki, an assistant professor at Purdue University’s School of Engineering Technology, another researcher who was not involved in the study. An all-organic device would be advantageous because it could be biocompatible—potentially allowing it to be implanted into the human body, for example. If organic neuromorphic devices reach that point, Nawrocki suggests, they may help in treating certain diseases and injuries to the nervous system. In the brain, he adds, neuromorphic implants could allow humans to control powered exoskeletons as well.
The organic neuromorphic chip also has the advantage of requiring less power than a standard chip. In order to switch, the organic transistors require only half a volt of electricity—about 20 times less than their silicon counterparts with similar dimensions, according to the authors of the new study. Because power is proportional to voltage, this means the entire system has lower power requirements. The neuromorphic chip is also relatively cheap to produce and comparatively simpler than a silicon system, van de Burgt says.
Such a low-power system could have many applications. For instance, it might help robots work for long hours at remote places on Earth—or even on another planet—without constantly needing to recharge, Krichmar says. Fifty or 100 years down the line, Nawrocki says, “we may have ultra-low-power autonomous robots, like artificial insects, that could even pollinate crops.”