I get goose bumps every time I see it. A paralyzed volunteer sits in a wheelchair while controlling a computer or robotic limb just with his or her thoughts—a demonstration of a brain-machine interface (BMI) in action.
That happened in my laboratory in 2013, when Erik Sorto, a victim of a gunshot wound when he was 21 years old, used his thoughts alone to drink a beer without help for the first time in more than 10 years. The BMI sent a neural message from a high-level cortical area. An electromechanical appendage was then able to reach out and grasp the bottle, raising it to Sorto’s lips before he took a sip. His drink came a year after surgery to implant electrodes in his brain to control signals that govern the thoughts that trigger motor movement. My lab colleagues and I watched in wonderment as he completed this deceptively simple task that is, in reality, intricately complex.
Witnessing such a feat immediately raises the question of how mere thoughts can control a mechanical prosthesis. We move our limbs unthinkingly every day—and completing these motions with ease is the goal of any sophisticated BMI. Neuroscientists, though, have tried for decades to decode neural signals that initiate movements to reach out and grab objects. Limited success in reading these signals has spurred a search for new ways to tap into the cacophony of electrical activity resonating as the brain’s 86 billion neurons communicate. A new generation of BMIs now holds the promise of creating a seamless tie between brain and prosthesis by tapping with great precision into the neural regions that formulate actions—whether the desired goal is grasping a cup or taking a step.
From Brain to Robot
A BMI operates by sending and receiving—“writing” and “reading”—messages to and from the brain. There are two major classes of the interface technology. A “write-in” BMI generally uses electrical stimulation to transmit a signal to neural tissue. Successful clinical applications of this technology are already in use. The cochlear prosthesis stimulates the auditory nerve to enable deaf subjects to hear. Deep-brain stimulation of an area that controls motor activity, the basal ganglia, treats motor disorders such as Parkinson’s disease and essential tremor. Devices that stimulate the retina are currently in clinical trials to alleviate certain forms of blindness.
“Read-out” BMIs, in contrast, record neural activity and are still at a developmental stage. The unique challenges of reading neural signals need to be addressed before this next-generation technology reaches patients. Coarse read-out techniques already exist. The electroencephalogram (EEG) records the average activity over centimeters of brain tissue, capturing the activity of many millions of neurons rather than that from individual neurons in a single circuit. Functional magnetic resonance imaging (fMRI) is an indirect measurement that records an increase in blood flow to an active region. It can image smaller areas than EEG, but its resolution is still rather low. Changes in blood flow are slow, so fMRI cannot distinguish rapid changes in brain activity.
To overcome these limitations, ideally one would like to record the activity of individual neurons. Observing changes in the firing rate of large numbers of single neurons can provide the most complete picture of what is happening in a specific brain region. In recent years arrays of tiny electrodes implanted in the brain have begun to make this type of recording possible. The arrays now in use are four-by-four-millimeter flat surfaces with 100 electrodes. Each electrode, measuring one to 1.5 millimeters long, sticks out of the flat surface. The entire array, which resembles a bed of nails, can record activity from 100 to 200 neurons.
The signals recorded by these electrodes move to “decoders” that use mathematical algorithms to translate varied patterns of single-neuron firing into a signal that initiates a particular movement, such as control of a robotic limb or a computer. These read-out BMIs will assist patients who have sustained brain injury because of spinal cord lesions, stroke, multiple sclerosis, amyotrophic lateral sclerosis and Duchenne muscular dystrophy.
Our lab has concentrated on people with tetraplegia, who are unable to move either their upper or lower limbs because of upper spinal cord injuries. We make recordings from the cerebral cortex, the approximately three-millimeter-thick surface of the brain’s two large hemispheres. If spread flat, the cortex of each hemisphere would measure about 80,000 square millimeters. The number of cortical regions that specialize in controlling specific brain functions has grown as more data have been collected and is now estimated to encompass more than 180 areas. These locations process sensory information, communicate to other brain regions involved with cognition, make decisions or send commands to trigger an action.
In short, a brain-machine interface can interact with many areas of the cortex. Among them are the primary cortical areas, which detect sensory inputs, such as the angle and intensity of light impinging on the retina or the sensation triggered in a peripheral nerve ending. Also targeted are the densely connected association cortices between the primary areas that are specialized for language, object recognition, emotion and executive control of decision-making.
A handful of groups have begun to record populations of single neurons in people who are paralyzed, allowing them to operate a prosthesis in the controlled setting of a lab. Major hurdles still persist before a patient can be outfitted with a neural prosthetic device as easily as a heart pacemaker. My group is pursuing recordings from the association areas instead of the motor cortex targeted by other labs. Doing so, we hope, may provide greater speed and versatility in sensing the firing of neural signals that convey one’s intentions.
The specific association area my lab has studied is the posterior parietal cortex (PPC), where plans to initiate movements begin. In our work with nonhuman primates, we found one subarea of the PPC, called the lateral intraparietal cortex, that discerns intentions to begin eye movements. Limb-movement processing occurs elsewhere in the PPC. The parietal reach region prepares arm movements. Also, Hideo Sakata, then at the Nihon University School of Medicine in Japan, and his colleagues found that the anterior intraparietal area formulates grasping movements.
Recordings from nonhuman primates indicate that the PPC provides several possible advantages for brain control of robotics or a computer cursor. It controls both arms, whereas the motor cortex in each hemisphere, the area targeted by other labs, activates primarily the limb on the opposite side of the body. The PPC also indicates the goal of a movement. When a nonhuman primate, for instance, is visually cued to reach for an object, this brain area switches on immediately, flagging the location of a desired object. In contrast, the motor cortex sends a signal for the path the reaching movement should take. Knowing the goal of an intended motor action lets the BMI decode it quickly, within a couple of hundred milliseconds, whereas figuring out the trajectory signal from the motor cortex can take more than a second.
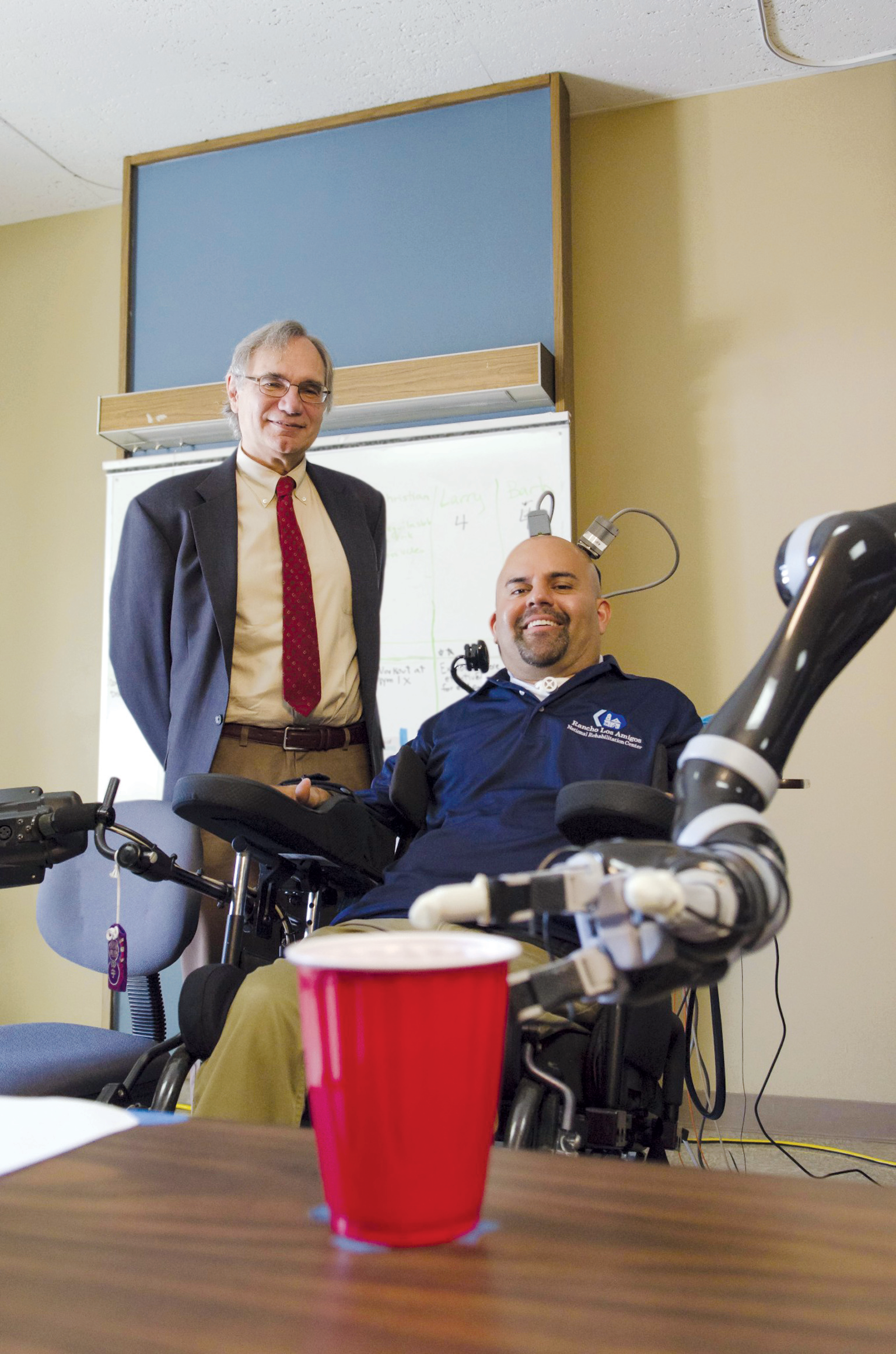
From Lab to Patient
It was not easy to go from experiments in lab animals to studies of the PPC in humans. Fifteen years elapsed before we made the first human implant. First, we inserted the same electrode arrays we planned to use in humans into healthy nonhuman primates. The monkeys then learned to control computer cursors or robotic limbs.
We built a team of scientists, clinicians and rehabilitation professionals from the California Institute of Technology, the University of Southern California, the University of California, Los Angeles, the Rancho Los Amigos National Rehabilitation Center, and Casa Colina Hospital and Centers for Healthcare. The team received a go-ahead from the Food and Drug Administration and institutional review boards charged with judging the safety and ethics of the procedure in the labs, hospitals and rehabilitation clinics involved.
A volunteer in this type of project is a true pioneer because he or she may or may not benefit. Participants ultimately join to help users of the technology who will seek it out once it is perfected for everyday use. The implant surgery for Sorto, our first volunteer, took place in April 2013 and was performed by neurosurgeons Charles Liu and Brian Lee. The procedure went flawlessly, but then came the wait for healing before we could test the device.
My colleagues at NASA’s Jet Propulsion Laboratory, which built and launched the Mars rovers, talk about the seven minutes of terror when a rover enters the planet’s atmosphere before it lands. For me it was two weeks of trepidation, wondering whether the implant would work. We knew in nonhuman primates how similar areas of the brain functioned, but a human implant was testing uncharted waters. No one had ever tried to record from a population of human PPC neurons before.
During the first day of testing we detected neural activity, and by the end of the week there were signals from enough neurons to begin to determine if Sorto could control a robot limb. Some of the neurons varied their activity when Sorto imagined rotating his hand. His first task consisted of turning the robot hand to different orientations to shake hands with a graduate student. He was thrilled, as were we, because this accomplishment marked the first time since his injury he could interact with the world using the bodily movement of a robotic arm.
People often ask how long it takes to learn to use a BMI. In fact, the technology worked right out of the box. It was intuitive and easy to use the brain’s intention signals to control the robotic arm. By imagining different actions, Sorto could watch recordings of individual neurons from his cortex and turn them on and off at will.
We ask participants at the beginning of a study what they would like to achieve by controlling a robot. For Sorto, he wanted to be able to drink a beer on his own rather than asking someone else for help. He was able to master this feat about one year into the study. With the team co-led by research scientist Spencer Kellis of Caltech, which included roboticists from the Applied Physics Laboratory at Johns Hopkins University, we melded Sorto’s intention signals with the processing power furnished by machine vision and smart robotic technology.
The vision algorithm analyzes inputs from video cameras, and the smart robot combines the intent signal with computer algorithms to initiate the movement of the robot arm. Sorto achieved this goal after a year’s time with cheers and shouts of joy from everyone present. In 2015 we published in Science our first results on using intention signals from the PPC to control neural prostheses.
Sorto is not the only user of our technology. Nancy Smith, now in her fourth year in the study, became tetraplegic from an automobile accident about 10 years ago. She had been a high school teacher of computer graphics and played piano as a pastime. In our studies with lead team members Tyson Aflalo of Caltech and Nader Pouratian of U.C.L.A., we found a detailed representation of the individual digits of both hands in Smith’s PPC. Using virtual reality, she could imagine and move 10 fingers individually on left and right “avatar” hands displayed on a computer screen. Using the imagined movement of five fingers from one hand, Smith could play simple melodies on a computer-generated piano keyboard.
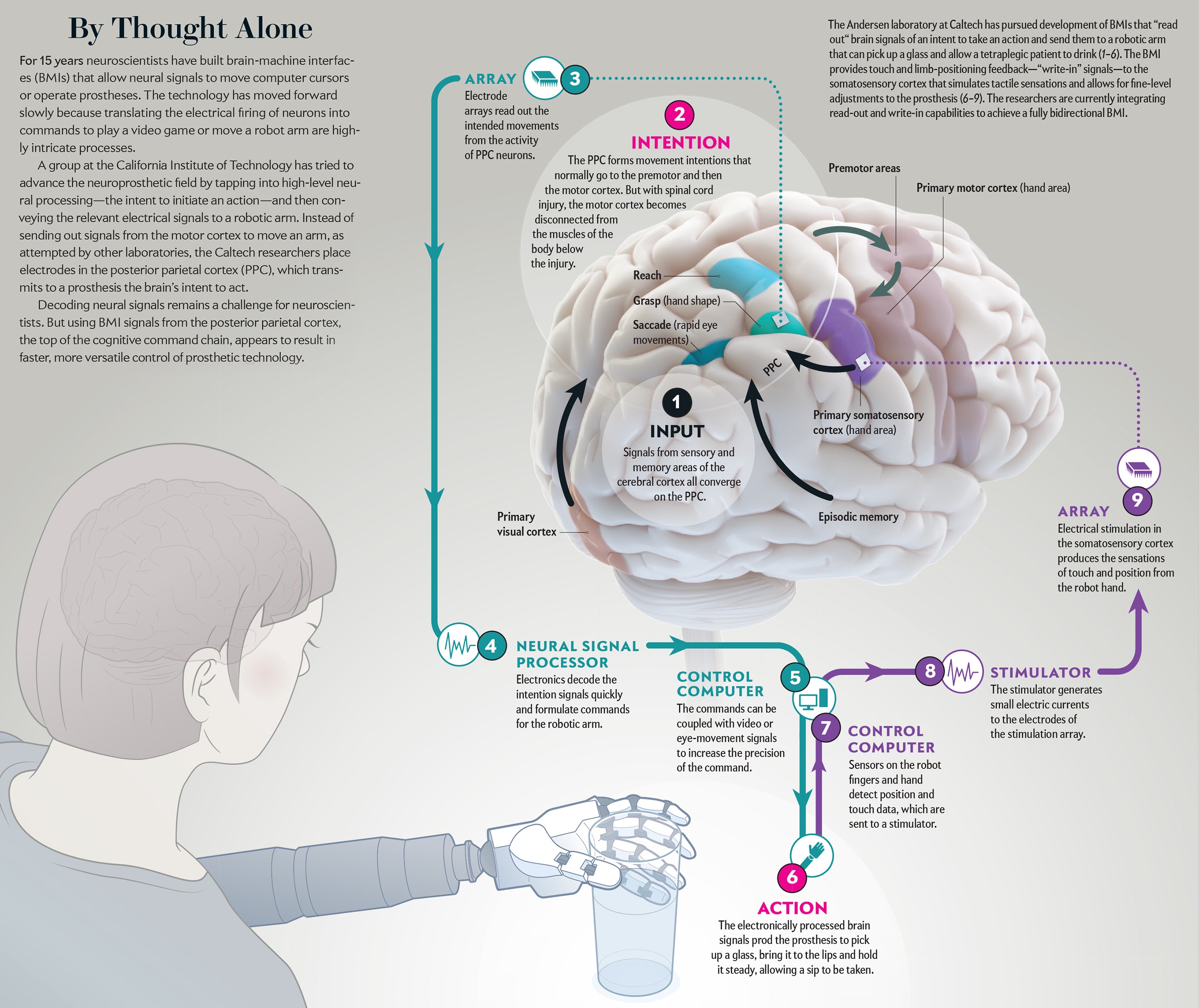
How the Brain Represents Goals
We were thrilled in working with these patients to find neurons tuned to processing signals related to one’s intentions. The amount of information to be gleaned from just a few hundred neurons turned out to be overwhelming. We could decode a range of cognitive activity, including mental strategizing (imagined versus attempted motion), finger movements, decisions about recalling visual stimuli, hand postures for grasping, observed actions, action verbs such as “grasp” or “push,” and visual and somatosensory perception. To our surprise, inserting a few tiny electrode arrays enabled us to decode much of what a person intends to do, as well as the sensory inputs that lead to the formation of intentions.
The question of how much information can be recorded from a small patch of brain tissue reminded me of a similar scientific problem that I had encountered early in my career. During my postdoctoral training with the late Vernon Mountcastle at the Johns Hopkins University School of Medicine, we examined how visual space is represented in the PPC of monkeys. Our eyes are like cameras, with the photosensitive retinas signaling the location of visual stimuli imaged on them—the entire image is referred to as a retinotopic map. Neurons respond to limited regions of the retina, referred to as their receptive fields. In other ways, processing visual perception is different than a video camera recording. When a video camera moves around, the recorded image also shifts, but when we move our eyes the world seems stable. The retinotopic image coming from the eyes must be converted into a visual representation of space that takes into account where the eyes are looking so that as they move, the world does not appear as if it were sliding around.
The PPC is a key processing center for high-order visual space representation. For a person to reach and grab an object, the brain needs to take into account where the eyes are looking to pick it up. PPC lesions in humans produce inaccurate reaching. In Mountcastle’s lab, we found individual PPC neurons had receptive fields that registered parts of a scene. The same cells also carried eye-position information. The two signals interacted by multiplying the visual response by the position of the eyes in the head—the product of which is called a gain field.
I continued to pursue this problem of understanding the brain’s representation of space when I took my first faculty position at the Salk Institute for Biological Studies, right across the street from the University of California, San Diego. Working with David Zipser, a U.C.S.D. theoretical neuroscientist developing neural networks, we reported in Nature on a computational model of a neural network that combined retinotopic locations with gaze direction to make maps of space that are invariant to eye movements. During training of the neural networks, their middle layers developed gain fields, just as was the case in the PPC experiments. By mixing signals for visual inputs and eye positions within the same neurons, as few as nine neurons could represent the entire visual field.
Recently this idea of mixed representations—populations of neurons responding to multiple variables (as with the gain fields)—has attracted renewed attention. For instance, recordings from the prefrontal cortex show a mixing of two types of memory task and different visual objects.
This work, moreover, may have a direct bearing in explaining what is happening in the PPC. We discovered this when we asked Smith, using a set of written instructions, to perform eight different combinations of a task. One of her undertakings required strategizing to imagine or attempt an action. Another necessitated using the right and left side of the body; a third entailed squeezing a hand or shrugging a shoulder. We found that PPC neurons mixed all these variables—and the intermingling exhibited a specific pattern, unlike the random interactions we and others had reported in lab animal experiments.
Activity of populations of neurons for strategizing and for controlling each body side tends to overlap. If a neuron fires to initiate the movement of the left hand, it will most likely also respond for an attempted righthand movement, whereas neuron groups that control the shoulder and hand are more separated. We refer to this type of representation as partially mixed selectivity. We have since found similarities in partially mixed representations that seem to make up a semantics of movement. The activity of cells tuned for the same action type tends to overlap. A neuron that responds to videos of a person grasping an object will also likely become active when a person reads the word “grasp.” But cells responding to an action such as pushing tend to get separated into their own group. In general, partially mixed coding appears to underlie computations that are similar (movements of the left hand are similar to those of the right). It also separates those that exhibit varying forms of neural processing (movement of the shoulder differs from movement of the hand).
Mixed and partially mixed coding have been found in certain parts of the association cortex—and new studies must explore whether they appear in other locations that govern language, object recognition and executive control. Additionally, we would like to know whether the primary sensory or motor cortical regions use a similar partially mixed structure.
Current studies indicate that, at least in the somatosensory cortex, neurons do not respond to visual stimuli or the intention to make a movement but do respond to somatosensory stimuli and to the imagined execution of movements. Thus, there is direct evidence that variables seen in the human PPC are not found in the primary somatosensory cortex, although it is still possible that partially mixed selectivity may exist in both areas but for different sets of variables.
Another near-future goal is to find out how much learning new tasks can affect the performance of the volunteers using the prosthesis. If learning readily takes place, any area of the brain might then be implanted and trained for any conceivable BMI task. For instance, an implant in the primary visual cortex could learn to control motor tasks. But if learning is more restricted, an implant would be needed in a motor area to perform motor tasks. Early results suggest this latter possibility, and an implant may have to be placed in the area that has been previously identified as controlling particular neural functions.
Writing in Sensations
A BMI must do more than just receive and process brain signals—it must also send feedback from a prosthesis to the brain. When we reach to pick up an object, visual feedback helps to direct the hand to the target. The positioning of the hand depends on the shape of the object to be grasped. If the hand does not receive touch and limb-positioning signals once it begins to manipulate the object, performance degrades quickly.
Finding a way to correct this deficit is critical for our volunteers with spinal cord lesions, who cannot move their body below the injury. They also do not perceive the tactile sensations or positioning of their body that are essential to fluid movement. An ideal neural prosthesis, then, must compensate through bidirectional signaling: it must transmit the intentions of the volunteer but also detect the touch and positioning information arriving from sensors on a robotic limb.
Robert Gaunt and his colleagues at the University of Pittsburgh have addressed this issue by implanting microelectrode arrays in the somatosensory cortex of a tetraplegic person—where inputs from the limbs process feelings of touch. Gaunt’s lab sent small electric currents through the microelectrodes, and the subject reported sensations from parts of the surface of the hand.
We have also used similar implants in the arm region of the somatosensory cortex. To our pleasant surprise, our subject, FG, reported natural sensations such as squeezing, tapping and vibrations on the skin, known as cutaneous sensations. He also perceived the feeling that the limb was moving—a sensation referred to as proprioception. These experiments show that subjects who have lost limb sensation can regain it through BMIs that have write-in perceptions. The next step is to provide a rich variety of somatosensory feedback sensations to improve robotic manual dexterity under brain control. Toward this goal, the Pittsburgh group has recently shown that stimulation of the primary somatosensory cortex improves the time to grasp objects with a robot limb, compared with standard visual feedback only. Also, we would like to know if subjects detect a sense of “embodiment,” in which the robot limb appears to become part of their body.
As these clinical studies show us, both writing in and reading out cortical signals, provide insight into the degree of reorganization of the cerebral cortex after neurological injury. Numerous studies have reported a high degree of reorganization, but until recently there has been little focus on the fundamental structure that remains intact. BMI studies show that tetraplegic subjects can quickly use the motor and the PPC cortex to control assistive devices, and stimulation of the somatosensory cortex produces sensations in deinnervated areas that are similar to what would be expected for intact individuals. These results demonstrate considerable stability of the adult cortex even after severe injury and in spite of injury-induced plasticity.
Future Challenges
A major future challenge is to develop better electrodes for sending and receiving neural signals. We have found that current implants continue to function for a relatively lengthy five years. But better electrodes would ideally push the longevity of these systems even further and increase the number of neurons that can be recorded from them. Another priority—an increase in the lengths of the electrodes’ tiny spikes—would help access areas located within folds of the cortex.
Flexible electrodes, which move with the slight jostling of the brain—from changes in blood pressure or the routine breathing cycle—will also allow for more stable recordings. Existing electrodes require recalibrating the decoder because the stiff electrodes change position with respect to neurons from day to day; researchers would ultimately like to follow the activity of identical neurons over weeks and months.
The implants need to be miniaturized, operate on low power (to avoid heating the brain), and function wirelessly so no cables are needed to connect the device to brain tissue. All current BMI technology needs to be implanted with a surgical procedure. But one day, we hope, recording and stimulation interfaces will be developed that can receive and send signals less invasively but with high precision. One step in this direction is our recent finding in nonhuman primates that ultrasound recorded changes in blood volume linked to neural activity can be used for BMIs. Because the skull is an impediment to ultrasound, a small ultrasound-transparent window would still be needed to replace a bit of the skull, but this surgery would be far less invasive than implanting microelectrode arrays that require opening the dura mater, the strong layer surrounding and protecting the brain, and directly inserting electrodes into the cortex.
BMIs, of course, are aimed at assisting people with paralysis. Yet science-fiction books, movies and the media have focused on the use of the technology for enhancement, conferring “superhuman” abilities that might allow a person to react faster, certainly an advantage for many motor tasks, or directly send and receive information from the cortex, much like having a small cell phone implanted in the brain. But enhancement is still very much in the realm of science fiction and will be achieved only when noninvasive technologies are developed that can operate at or near the precision of current microelectrode array technology.
Finally, I would like to convey the satisfaction of doing basic research and making it available to patients. Fundamental science is necessary to both advance knowledge and develop medical therapies. To be able to then transfer these discoveries into a clinical setting brings the research endeavor to its ultimate realization. A scientist is left with an undeniable feeling of personal fulfillment in sharing with patients their delight at being able to move a robotic limb to interact again with the physical world.