Human faces pop up on a screen, hundreds of them, one after another. Some have their eyes stretched wide, others show lips clenched. Some have eyes squeezed shut, cheeks lifted and mouths agape. For each one, you must answer this simple question: is this the face of someone having an orgasm or experiencing sudden pain?
Psychologist Rachael Jack and her colleagues recruited 80 people to take this test as part of a study in 2018. The team, at the University of Glasgow, UK, enlisted participants from Western and East Asian cultures to explore a long-standing and highly charged question: do facial expressions reliably communicate emotions?
Researchers have been asking people what emotions they perceive in faces for decades. They have questioned adults and children in different countries and Indigenous populations in remote parts of the world. Influential observations in the 1960s and 1970s by US psychologist Paul Ekman suggested that, around the world, humans could reliably infer emotional states from expressions on faces—implying that emotional expressions are universal.
These ideas stood largely unchallenged for a generation. But a new cohort of psychologists and cognitive scientists has been revisiting those data and questioning the conclusions. Many researchers now think that the picture is a lot more complicated, and that facial expressions vary widely between contexts and cultures. Jack’s study, for instance, found that although Westerners and East Asians had similar concepts of how faces display pain, they had different ideas about expressions of pleasure.
Researchers are increasingly split over the validity of Ekman’s conclusions. But the debate hasn’t stopped companies and governments accepting his assertion that the face is an emotion oracle—and using it in ways that are affecting people’s lives. In many legal systems in the West, for example, reading the emotions of a defendant forms part of a fair trial. As US Supreme Court judge Anthony Kennedy wrote in 1992, doing so is necessary to “know the heart and mind of the offender”.
Decoding emotions is also at the core of a controversial training programme designed by Ekman for the US Transportation Security Administration (TSA) and introduced in 2007. The programme, called SPOT (Screening Passengers by Observation Techniques), was created to teach TSA personnel how to monitor passengers for dozens of potentially suspicious signs that can indicate stress, deception or fear. But it has been widely criticized by scientists, members of the US Congress and organizations such as the American Civil Liberties Union for being inaccurate and racially biased.
Such concerns haven’t stopped leading tech companies running with the idea that emotions can be detected readily, and some firms have created software to do just that. The systems are being trialled or marketed for assessing the suitability of job candidates, detecting lies, making adverts more alluring and diagnosing disorders from dementia to depression. Estimates place the industry’s value at tens of billions of dollars. Tech giants including Microsoft, IBM and Amazon, as well as more specialist companies such as Affectiva in Boston, Massachusetts, and NeuroData Lab in Miami, Florida, all offer algorithms designed to detect a person’s emotions from their face.
With researchers still wrangling over whether people can produce or perceive emotional expressions with fidelity, many in the field think efforts to get computers to do it automatically are premature—especially when the technology could have damaging repercussions. The AI Now Institute, a research centre at New York University, has even called for a ban on uses of emotion-recognition technology in sensitive situations, such as recruitment or law enforcement.
Facial expressions are extremely difficult to interpret, even for people, says Aleix Martinez, who researches the topic at the Ohio State University in Columbus. With that in mind, he says, and given the trend towards automation, “we should be very concerned”.
Skin deep
The human face has 43 muscles, which can stretch, lift and contort it into dozens of expressions. Despite this vast range of movement, scientists have long held that certain expressions convey specific emotions.
One person who pushed this view was Charles Darwin. His 1859 book On the Origin of Species, the result of painstaking fieldwork, was a masterclass in observation. His second most influential work, The Expression of the Emotions in Man and Animals (1872), was more dogmatic.
Darwin noted that primates make facial movements that look like human expressions of emotion, such as disgust or fear, and argued that the expressions must have some adaptive function. For example, curling the lip, wrinkling the nose and narrowing the eyes—an expression linked to disgust—might have originated to protect the individual against noxious pathogens. Only as social behaviours started to develop, did these facial expressions take on a more communicative role.
The first cross-cultural field studies, carried out by Ekman in the 1960s, backed up this hypothesis. He tested the expression and perception of six key emotions—happiness, sadness, anger, fear, surprise and disgust—around the world, including in a remote population in New Guinea.
Ekman chose these six expressions for practical reasons, he told Nature. Some emotions, such as shame or guilt, do not have obvious readouts, he says. “The six emotions that I focused on do have expressions, which meant that they were amenable to study.”
Those early studies, Ekman says, showed evidence of the universality that Darwin’s evolution theory expected. And later work supported the claim that some facial expressions might confer an adaptive advantage.
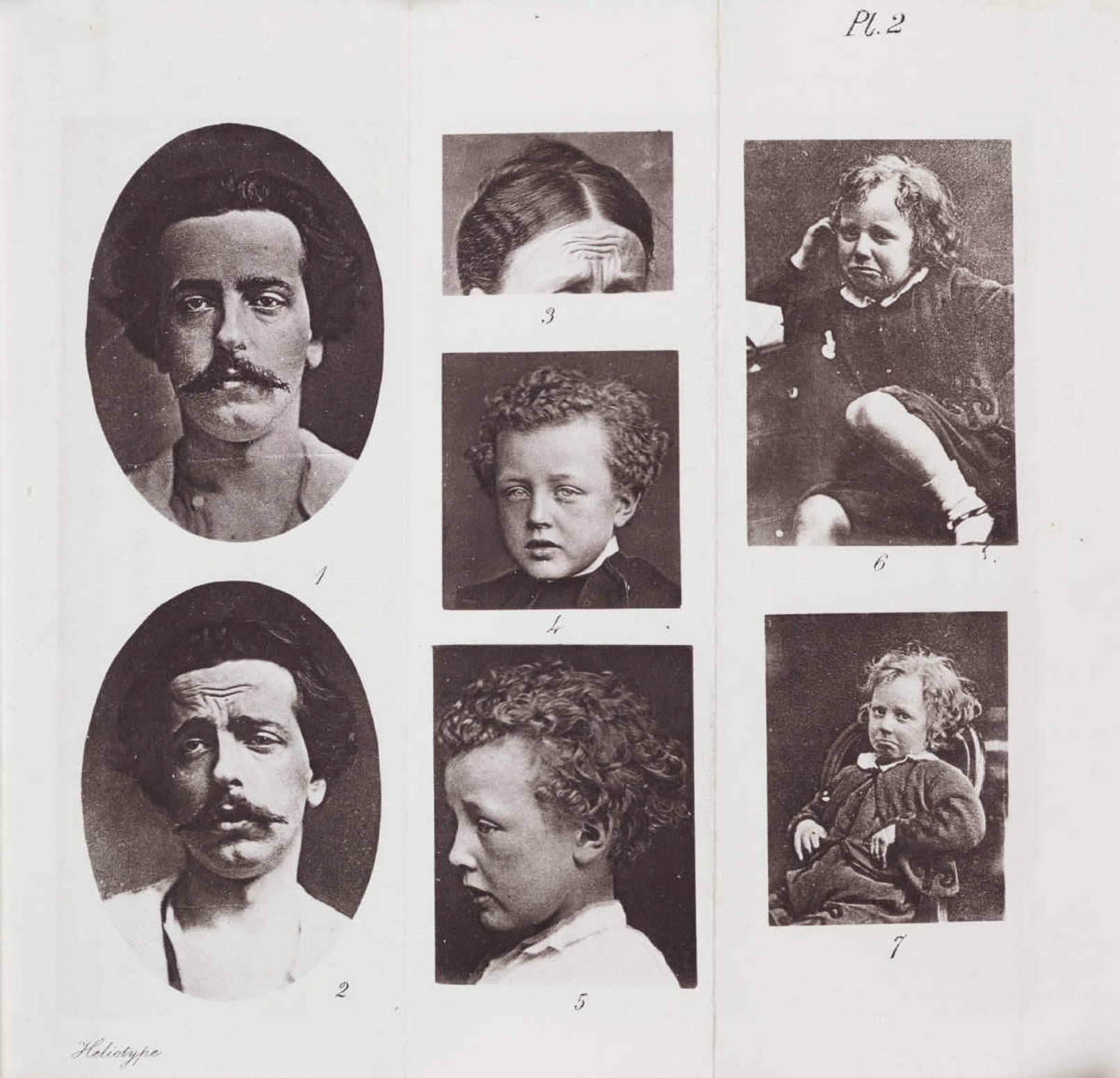
“The assumption for a long time was that facial expressions were obligatory movements,” says Lisa Feldman Barrett, a psychologist at Northeastern University in Boston who studies emotion. In other words, our faces are powerless to hide our emotions. The obvious problem with that assumption is that people can fake emotions, and can experience feelings without moving their faces. Researchers in the Ekman camp acknowledge that there can be considerable variation in the ‘gold standard’ expressions expected for each emotion.
But a growing crowd of researchers argues that the variation is so extensive that it stretches the gold-standard idea to the breaking point. Their views are backed up by a vast literature review. A few years ago, the editors of the journal Psychological Science in the Public Interest put together a panel of authors who disagreed with one another and asked them to review the literature.
“We did our best to set aside our priors,” says Barrett, who led the team. Instead of starting with a hypothesis, they waded into the data. “When there was a disagreement, we just broadened our search for evidence.” They ended up reading around 1,000 papers. After two and a half years, the team reached a stark conclusion: there was little to no evidence that people can reliably infer someone else’s emotional state from a set of facial movements.
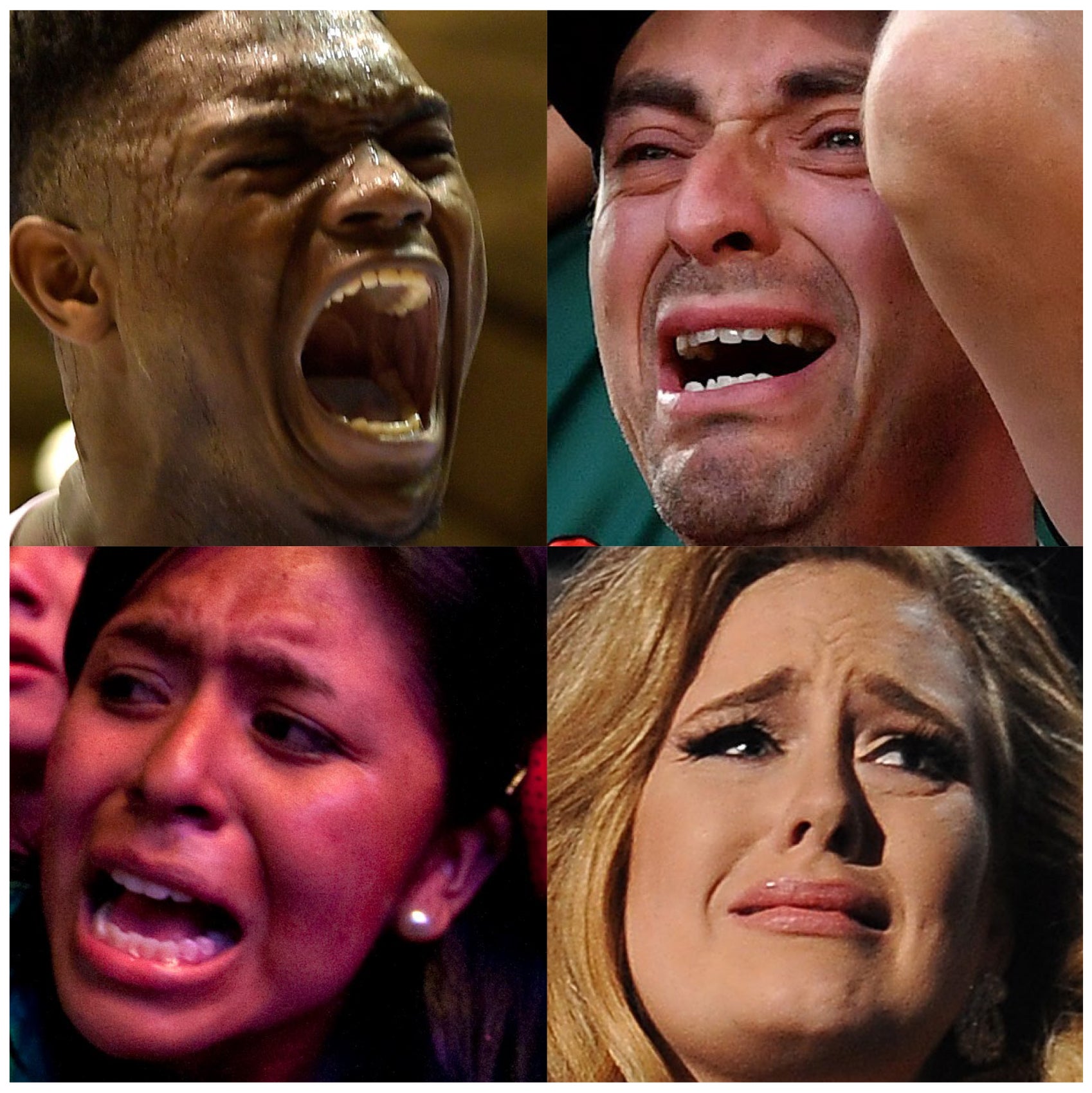
At one extreme, the group cited studies that found no clear link between the movements of a face and an internal emotional state. Psychologist Carlos Crivelli at De Montfort University in Leicester, UK, has worked with residents of the Trobriand islands in Papua New Guinea and found no evidence for Ekman’s conclusions in his studies. Trying to assess internal mental states from external markers is like trying to measure mass in metres, Crivelli concludes.
Another reason for the lack of evidence for universal expressions is that the face is not the whole picture. Other things, including body movement, personality, tone of voice and changes in skin tone have important roles in how we perceive and display emotion. For example, changes in emotional state can affect blood flow, and this in turn can alter the appearance of the skin. Martinez and his colleagues have shown that people are able to connect changes in skin tone to emotions. The visual context, such as the background scene, can also provide clues to someone’s emotional state.
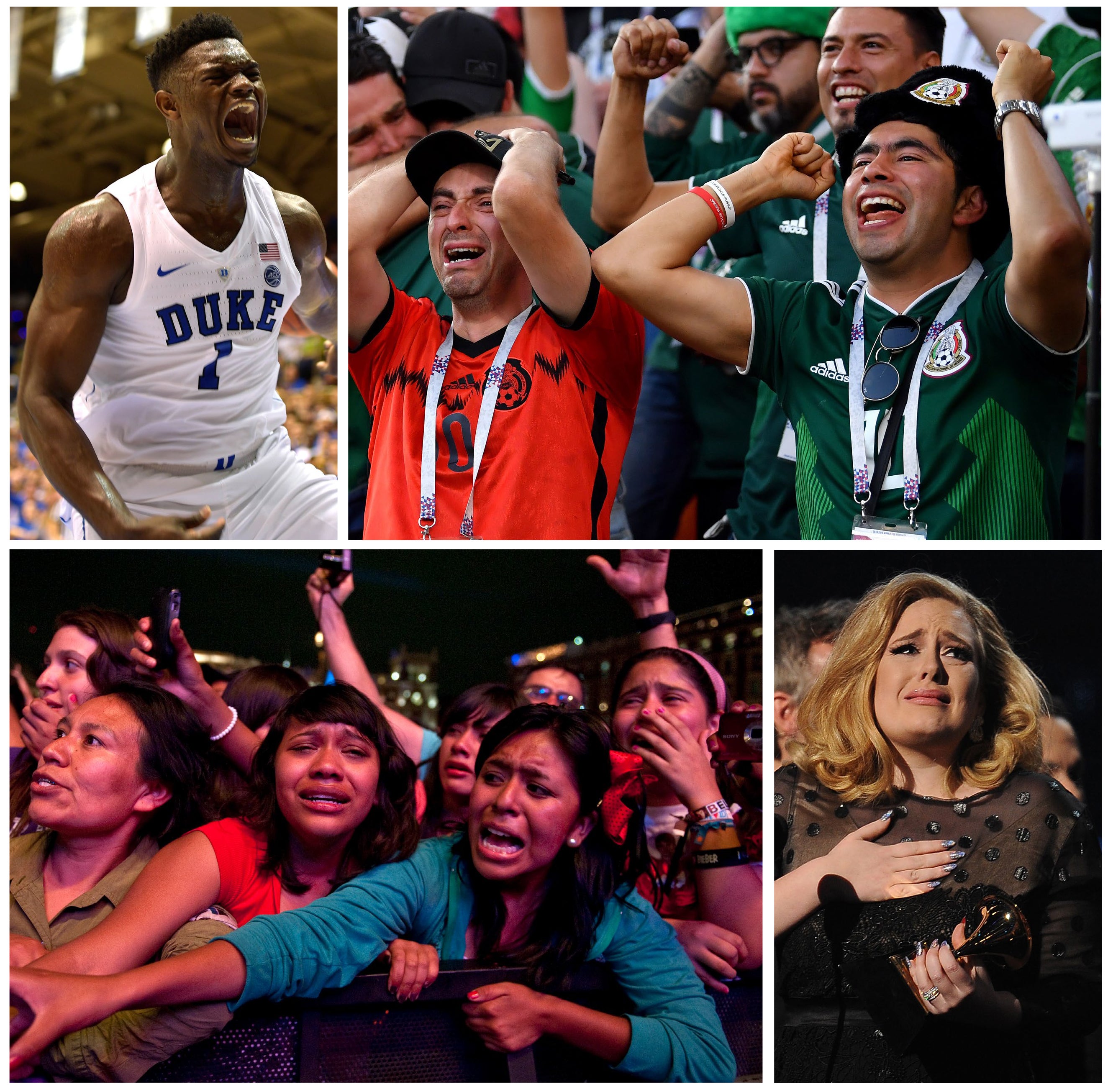
Mixed emotions
Other researchers think the push-back on Ekman’s results is a little overzealous—not least Ekman himself. In 2014, responding to a critique from Barrett, he pointed to a body of work that he says supports his previous conclusions, including studies on facial expressions that people make spontaneously, and research on the link between expressions and underlying brain and bodily state. This work, he wrote, suggests that facial expressions are informative not only about individuals’ feelings, but also about patterns of neurophysiological activation (see go.nature.com/2pmrjkh). His views have not changed, he says.
According to Jessica Tracy, a psychologist at the University of British Columbia in Vancouver, Canada, researchers who conclude that Ekman’s theory of universality is wrong on the basis of a handful of counterexamples are overstating their case. One population or culture with a slightly different idea of what makes an angry face doesn’t demolish the whole theory, she says. Most people recognize an angry face when they see it, she adds, citing an analysis of nearly 100 studies. “Tons of other evidence suggests that most people in most cultures all over the world do see this expression is universal.”
Tracy and three other psychologists argue that Barrett’s literature review caricatures their position as a rigid one-to-one mapping between six emotions and their facial movements. “I don’t know any researcher in the field of emotion science who thinks this is the case,” says Disa Sauter at the University of Amsterdam, a co-author of the reply.
Sauter and Tracy think that what is needed to make sense of facial expressions is a much richer taxonomy of emotions. Rather than considering happiness as a single emotion, researchers should separate emotional categories into their components; the happiness umbrella covers joy, pleasure, compassion, pride and so on. Expressions for each might differ or overlap.
At the heart of the debate is what counts as significant. In a study in which participants choose one of six emotion labels for each face they see, some researchers might consider that an option that is picked more than 20% of the time shows significant commonality. Others might think 20% falls far short. Jack argues that Ekman’s threshold was much too low. She read his early papers as a PhD student. “I kept going to my supervisor and showing him these charts from the 1960s and 1970s and every single one of them shows massive differences in cultural recognition,” she says. “There’s still no data to show that emotions are universally recognized.”
Significance aside, researchers also have to battle with subjectivity: many studies rely on the experimenter having labelled an emotion at the start of the test, so that the end results can be compared. So Barrett, Jack and others are trying to find more neutral ways to study emotions. Barrett is looking at physiological measures, hoping to provide a proxy for anger, fear or joy. Instead of using posed photographs, Jack uses a computer to randomly generate facial expressions, to avoid fixating on the common six. Others are asking participants to group faces into as many categories as they think are needed to capture the emotions, or getting participants from different cultures to label pictures in their own language.
In silico sentiment
Software firms tend not to allow their algorithms such scope for free association. A typical artificial intelligence (AI) program for emotion detection is fed millions of images of faces and hundreds of hours of video footage in which each emotion has been labelled, and from which it can discern patterns. Affectiva says it has trained its software on more than 7 million faces from 87 countries, and that this gives it an accuracy in the 90th percentile. The company declined to comment on the science underlying its algorithm. Neurodata Lab acknowledges that there is variation in how faces express emotion, but says that “when a person is having an emotional episode, some facial configurations occur more often than a chance would allow”, and that its algorithms take this commonality into account. Researchers on both sides of the debate are sceptical of this kind of software, however, citing concerns over the data used to train algorithms and the fact that the science is still debated.
Ekman says he has challenged the firms’ claims directly. He has written to several companies—he won’t reveal which, only that “they are among the biggest software companies in the world”—asking to see evidence that their automated techniques work. He has not heard back. “As far as I know, they’re making claims for things that there is no evidence for,” he says.
Martinez concedes that automated emotion detection might be able to say something about the average emotional response of a group. Affectiva, for example, sells software to marketing agencies and brands to help predict how a customer base might react to a product or marketing campaign.
If this software makes a mistake, the stakes are low—an advert might be slightly less effective than hoped. But some algorithms are being used in processes that could have a big impact on people’s lives, such as in job interviews and at borders. Last year, Hungary, Latvia and Greece piloted a system for prescreening travellers that aims to detect deception by analysing microexpressions in the face.
Settling the emotional-expressions debate will require different kinds of investigation. Barrett—who is often asked to present her research to technology companies, and who visited Microsoft this month—thinks that researchers need to do what Darwin did for On the Origin of Species: “Observe, observe, observe.” Watch what people actually do with their faces and their bodies in real life—not just in the lab. Then use machines to record and analyse real-world footage.
Barrett thinks that more data and analytical techniques could help researchers to learn something new, instead of revisiting tired data sets and experiments. She throws down a challenge to the tech companies eager to exploit what she and many others increasingly see as shaky science. “We’re really at this precipice,” she says. “Are AI companies going to continue to use flawed assumptions or are they going do what needs to be done?”
This article is reproduced with permission and was first published on February 26 2020.