The Physician
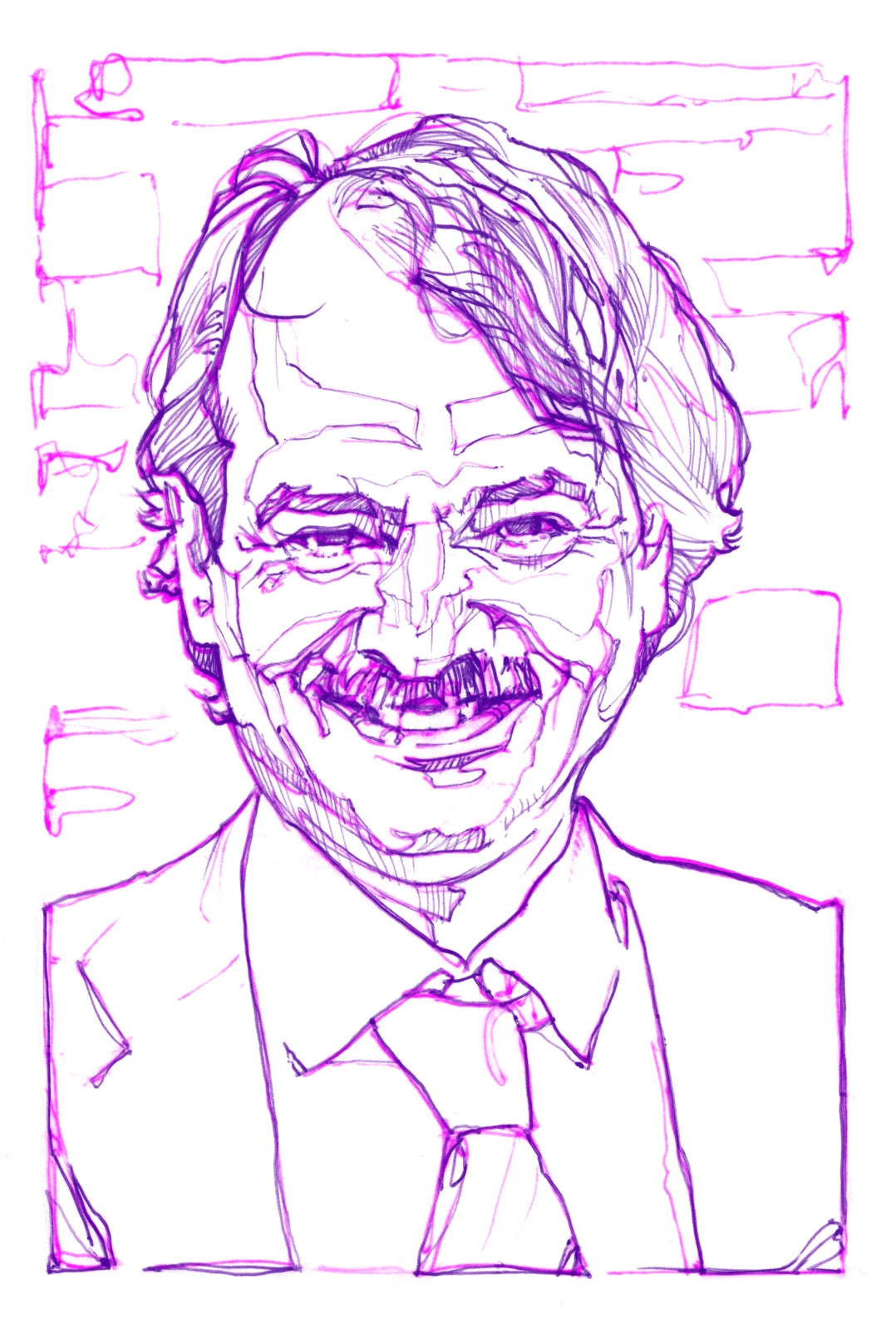
The answer to questions about human life isn't a certain thing, like measuring how a stone drops to the ground in exactly so many seconds. If it were, it probably would not be life. It would be a stone. Within biomedicine, it's tricky finding out if an effect is real—there are different standards across different fields. Not all tools will work for every question, and there are different levels of complexity for what we know before we even start a study.
Still, the one core dimension across biomedicine is the ability to replicate, in a new study, what was seen in the first investigation. For many years in the field, we have been discouraged from doing this. Why waste money to do the exact thing you had done before, let alone something someone else had done before? But many researchers are realizing it is not possible to leave out replication studies.
To make replication work, though, it is essential to have a detailed explanation of how the original study was done. You need the instructions, the raw data and maybe even some custom-built computer software. For a long time, scientists didn't want to share that information, but that is changing. Science is a communal effort, and we should default to being open and sharing.
The Historical Linguist
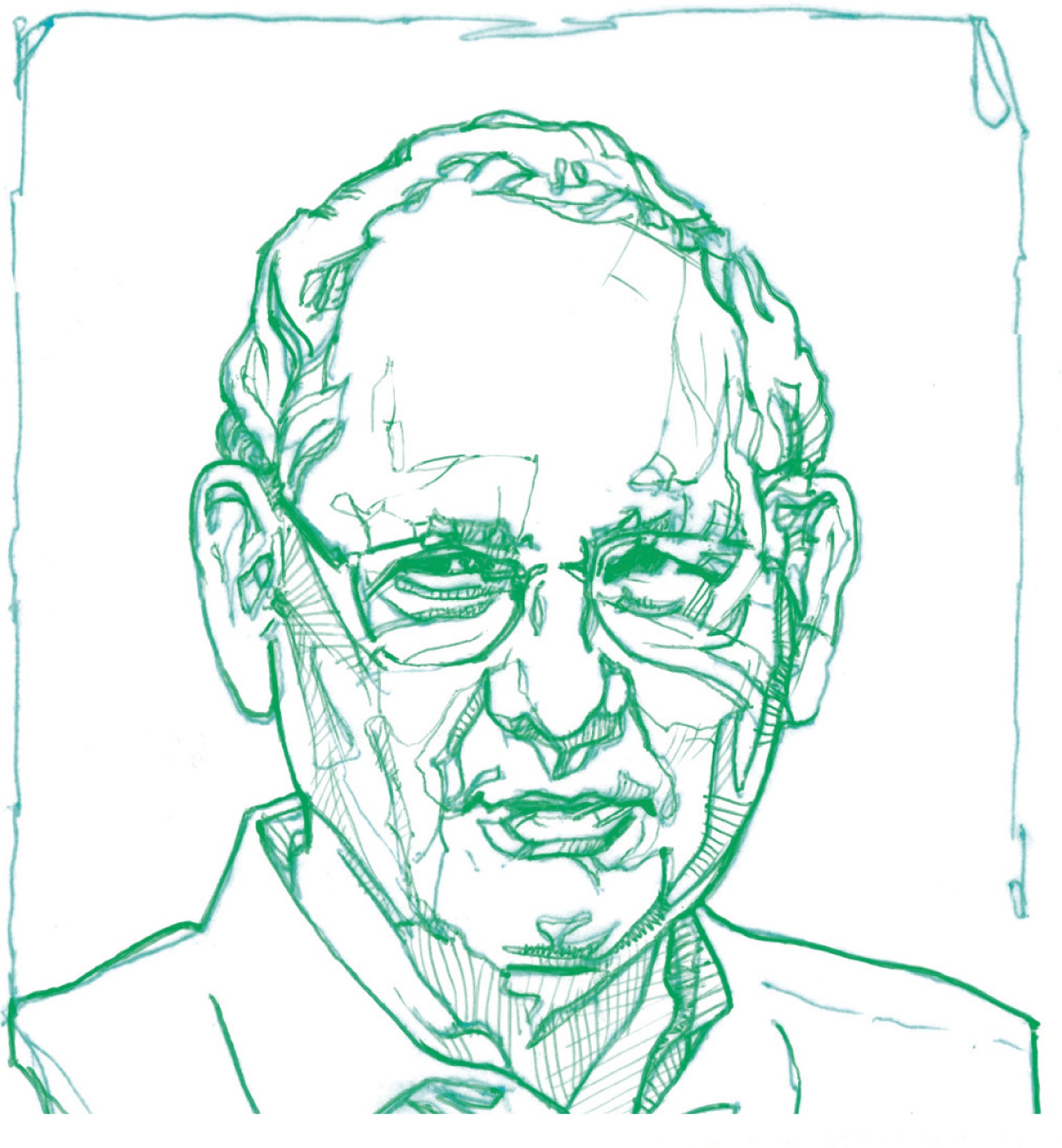
Like any scientist, linguists rely on the scientific method. One of the principal goals of linguistics is to describe and analyze languages to discover the full range of what is possible and not possible in human languages. From this, linguists aim to reach their goal of understanding human cognition through the capacity for human language.
So there is an urgency to efforts to describe endangered languages, to document them while they are still in use, to determine the full range of what is linguistically possible. There are around 6,500 known human languages; around 45 percent of them are endangered.
Linguists use a specific set of criteria to identify endangered languages and to determine just how endangered a language is: Are children still learning the language? How many individual people speak it? Is the percentage of speakers declining with respect to the broader population? And are the contexts in which the language is used decreasing?
The question of scientific objectivity and “truth” is connected to endangered language research. Truth, in a way, is contextual. That is, what we hold to be true can change as we get more data and evidence or as our methods improve. The investigation of endangered languages often discovers things that we did not know were possible in languages, forcing us to reexamine previous claims about the limits of human language, so that sometimes what we thought was true can shift.
The Paleobiologist
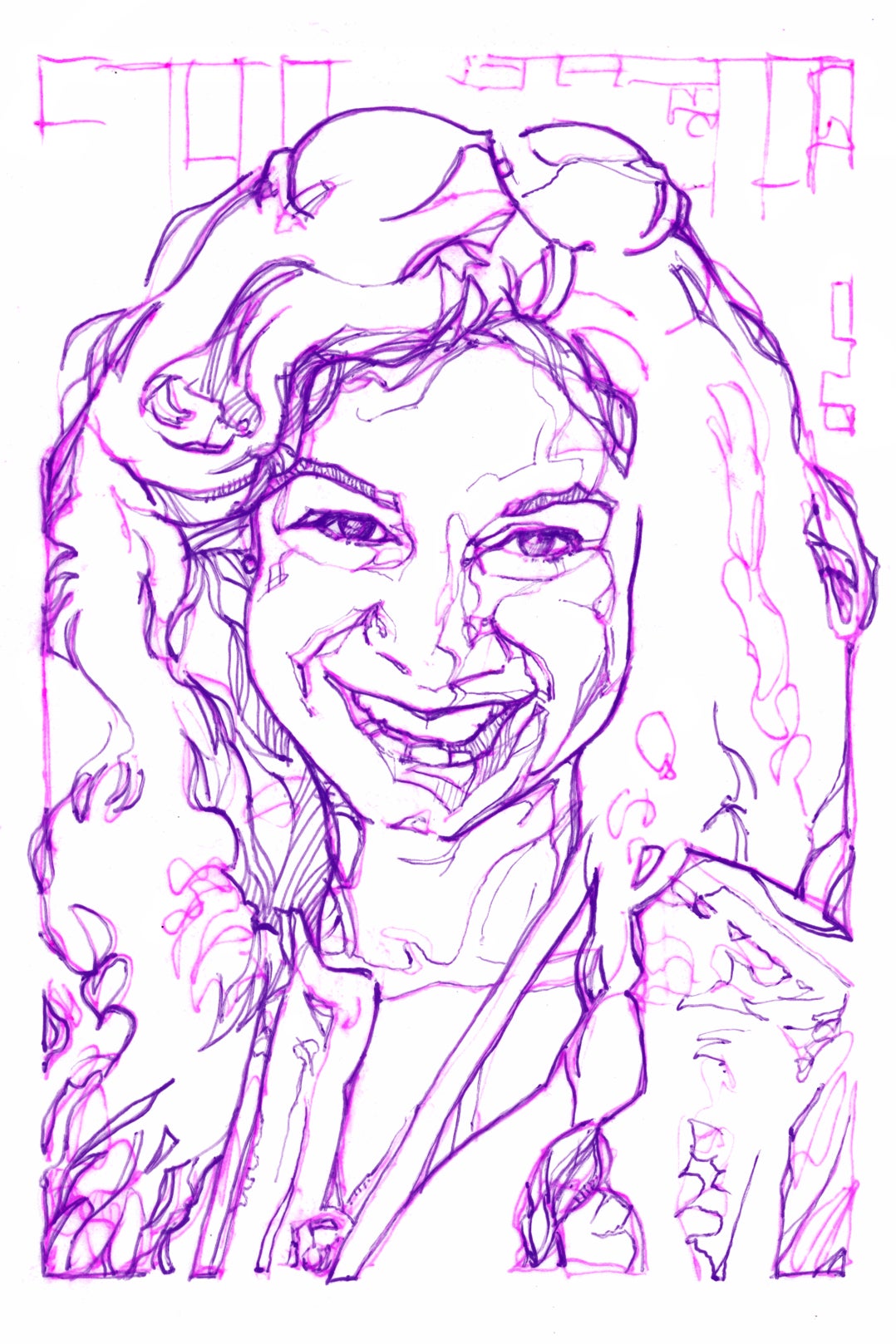
Our basic unit of truth in paleobiology is the fossil—a clear record of life in the past—and we also use genetic evidence from living organisms to help us put fossils within the tree of life. Together they help us understand how these creatures changed and how they are related. Because we are looking at extinct animals as they existed in a broader ecosystem, we pull in information from other fields: chemical analysis of surrounding rocks to get a sense of the fossil’s age, where the world’s landmasses might have been at the time, what kind of environmental changes were happening, and so on.
To discover fossils, we scour the landscape to find them among rocks. You can tell the difference between a fossil and any old rock by its shape and its internal structure. For example, a fossil bone will have tiny cylinders called osteons where blood vessels once ran through the bone. Some fossils are obvious: a leg of a dinosaur, a giant, complete bone. Smaller bits can be telling, too. For mammals, which I study, you can tell a lot from the shape of a single tooth. And we can combine this information with genetics, by using DNA samples from living creatures that we think are related to the fossils, based on anatomy and other clues.
We just don’t do these investigations to reconstruct past worlds but also to see what they can tell us about our current world. There was a huge spike in temperature 55 million years ago, for example. It was nothing like today, but still, we’ve found radical changes in the animals and plants from that era. We can compare those changes to see how related creatures may respond to current climate change.
The Social Technologist
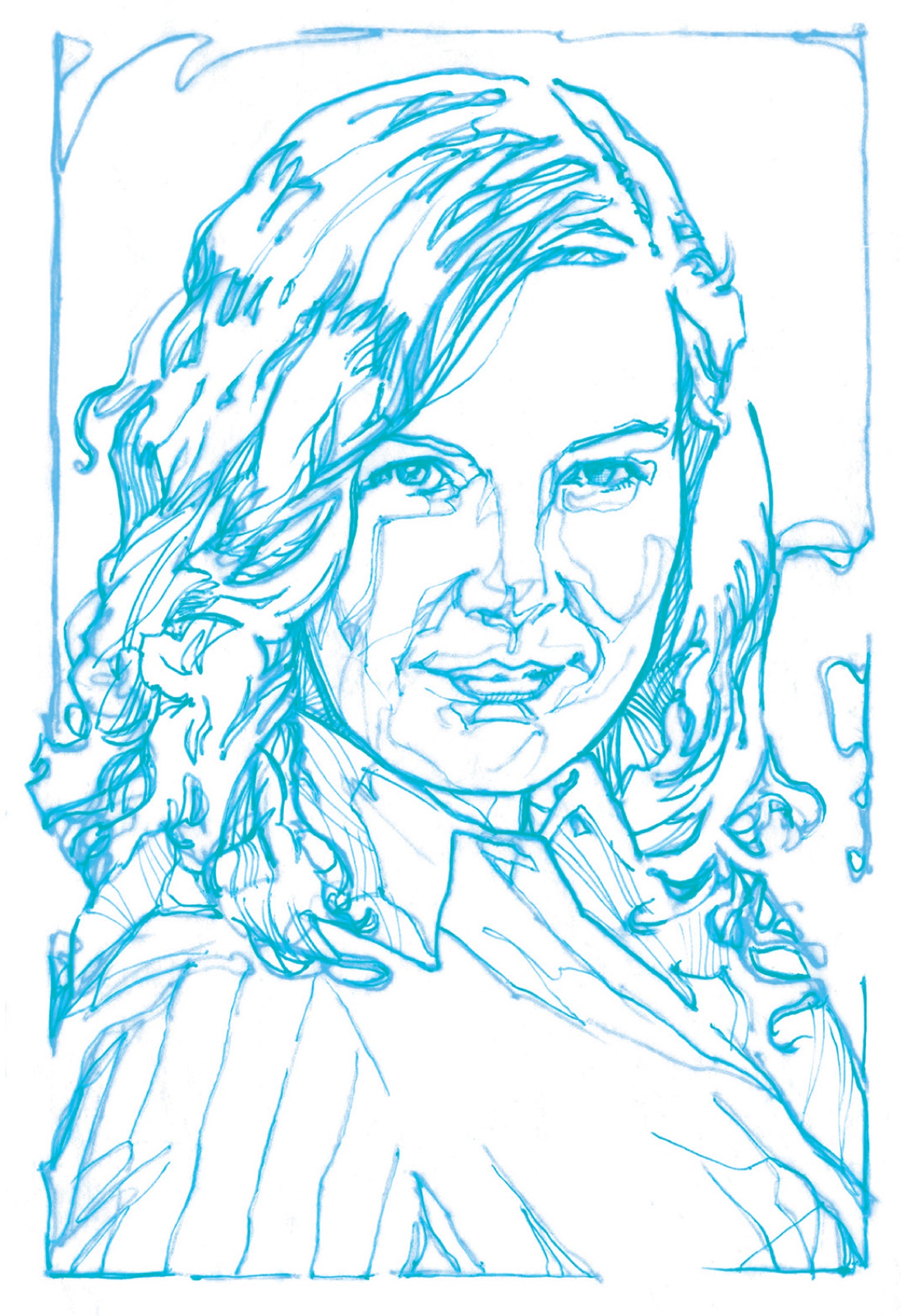
The biggest epistemological question facing the field of machine learning is: What is our ability to test a hypothesis? Algorithms learn to detect patterns and details from massive sets of examples—for instance, an algorithm could learn to identify a cat after seeing thousands of cat photographs. Until we have greater interpretability, we can test how a result was achieved by appealing conclusions from the algorithms. This raises the specter that we don't have real accountability for the results of deep-learning systems—let alone due process when it comes to their effects on social institutions. These issues are part of a live debate in the field.
Also, does machine learning represent a type of rejection of the scientific method, which aims to find not only correlation but also causation? In many machine-learning studies, correlation has become the new article of faith, at the cost of causation. That raises real questions about verifiability.
In some cases, we may be taking a step backward. We see this in the space of machine vision and affect recognition. These are systems that extrapolate from photographs of people to predict their race, gender, sexuality or likelihood of being a criminal. These sorts of approaches are both scientifically and ethically concerning—with echoes of phrenology and physiognomy. The focus on correlation should raise deep suspicions in terms of our ability to make claims about people's identity. That's a strong statement, by the way, but given the decades of research on these issues in the humanities and social sciences, it should not be controversial.
The Statistician
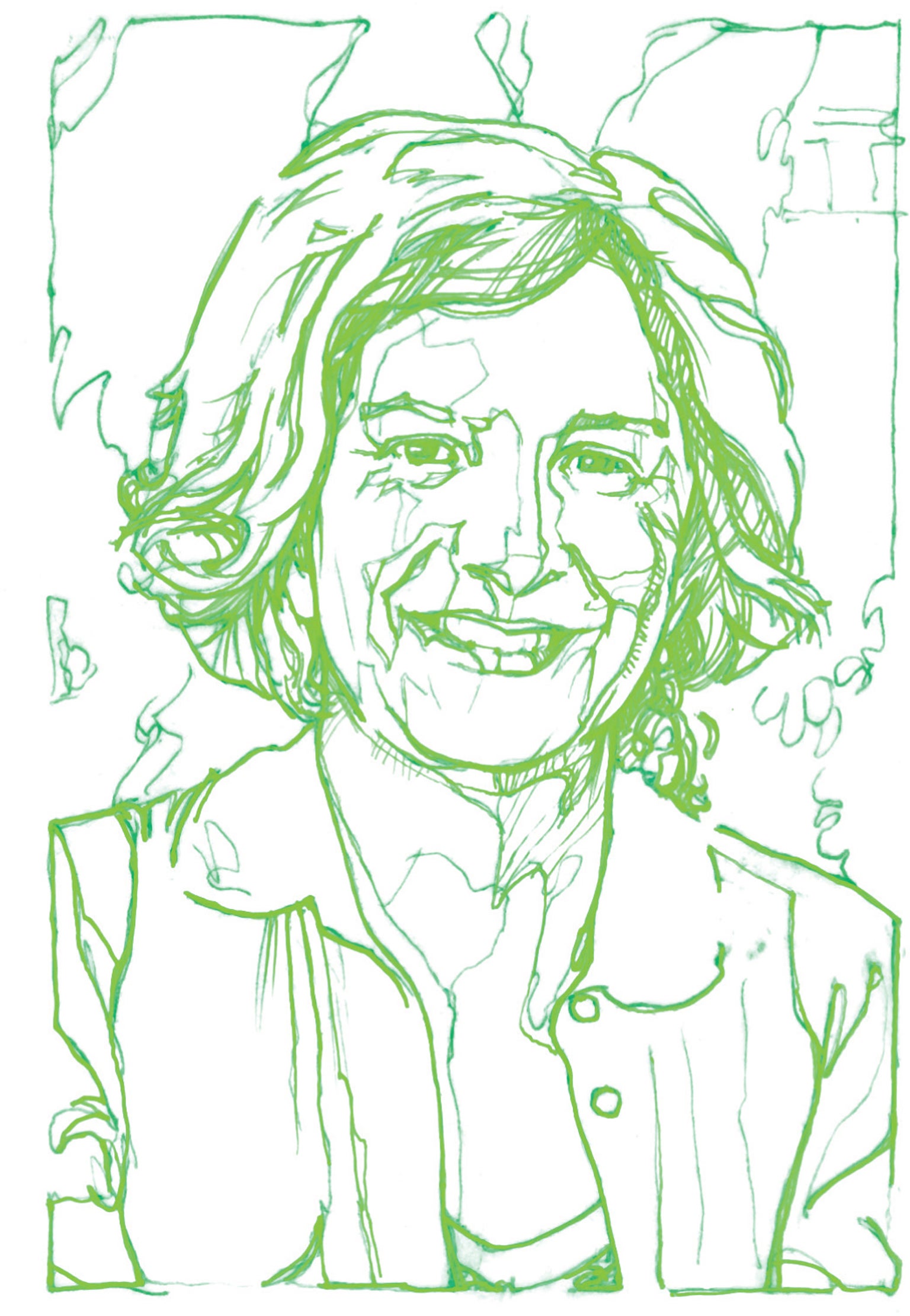
In statistics, we aren't generally seeing the whole universe but only a slice of it. A small slice usually, which could tell a completely different story than another small slice. We are trying to make a leap from these small slices to a bigger truth. A lot of people take that basic unit of truth to be the p-value, a statistical measure of how surprising what we see in our small slice is, if our assumptions about the larger universe hold. But I don't think that's correct.
In reality, the notion of statistical significance is based on an arbitrary threshold applied to the p-value, and it may have very little to do with substantive or scientific significance. It's too easy to slip into a thought pattern that provides that arbitrary threshold with meaning—it gives us a false sense of certainty. And it's also too easy to hide a multitude of scientific sins behind that p-value.
One way to strengthen the p-value would be to shift the culture toward transparency. If we not only report the p-value but also show the work on how we got there—the standard error, the standard deviation or other measures of uncertainty, for example—we can give a better sense of what that number means. The more information we publish, the harder it is to hide behind that p-value. Whether we can get there, I don't know. But I think we should try.
The Data Journalist
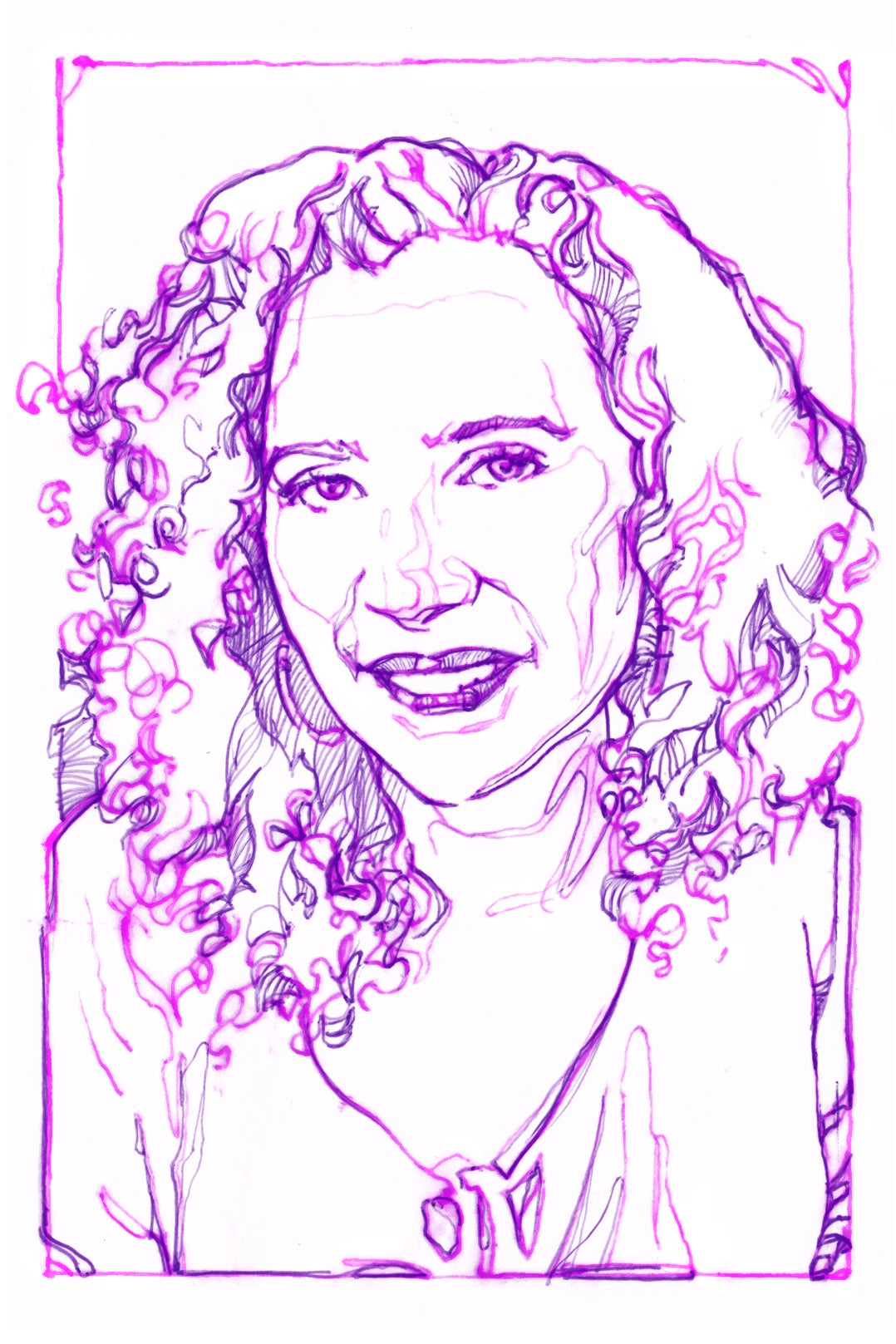
People assume that because there are data, that the data must be true. But the truth is, all data are dirty. People create data, which means data have flaws just like people. One thing data journalists do is interrogate that assumption of truth, which serves an important accountability function—a power check to make sure we aren't collectively getting carried away with data and making bad social decisions.
To interrogate the data, you have to do a lot of janitorial work. You have to clean and organize them; you have to check the math. And you also have to acknowledge the uncertainty. If you are a scientist, and you don't have the data, you can't write the paper. But one of the fabulous things about being a data journalist is that sparse data don't deter us—sometimes the lack of data tells me something just as interesting. As a journalist, I can use words, which are a magnificent tool for communicating about uncertainty.
The Behavioral Scientist
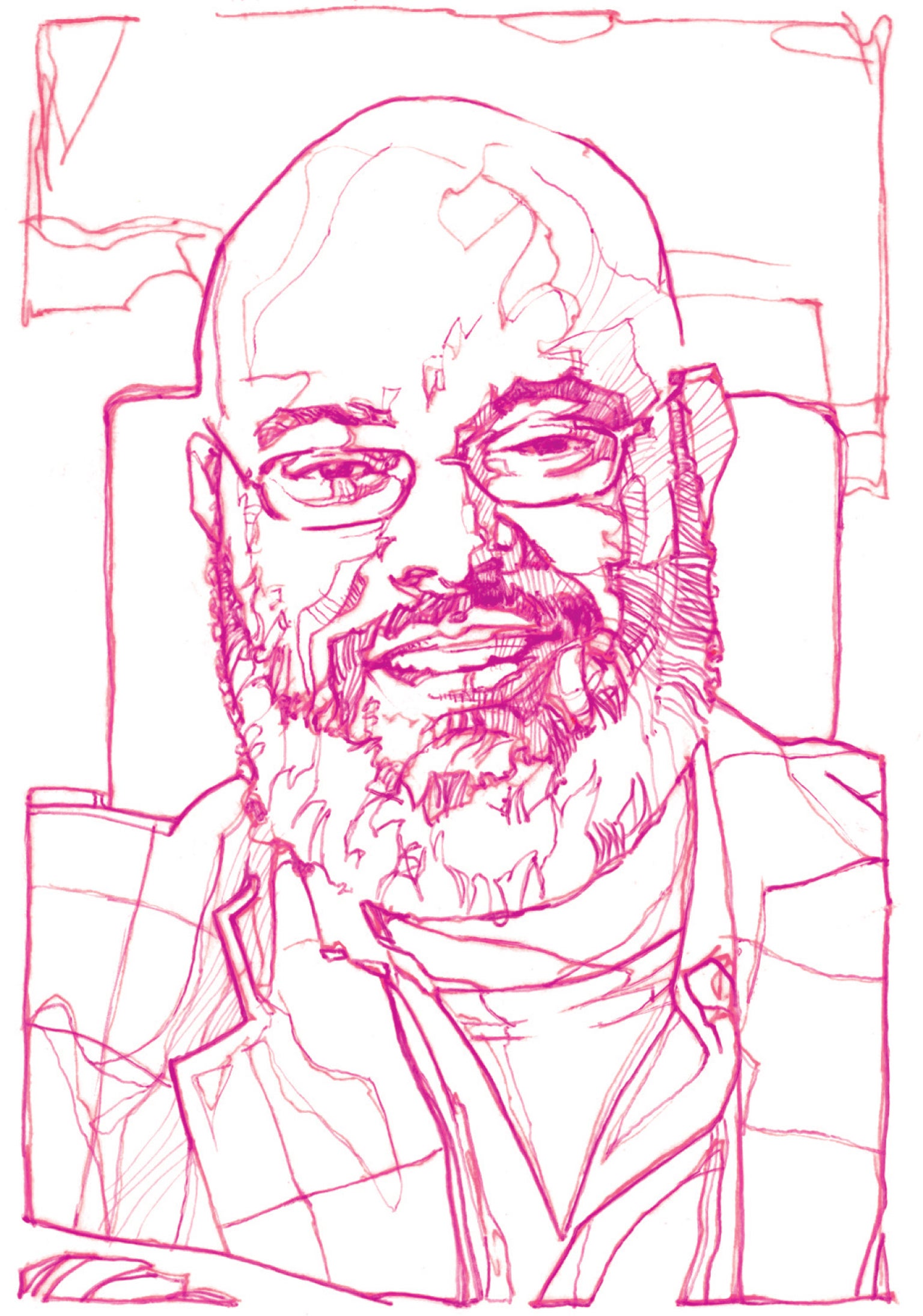
The kind of control you have in bench science is much tighter than in behavioral science—the power to detect small effects in people is much lower than in, say, chemistry. Not only that, people's behaviors change across time and culture. When we think about truth in behavioral science, it's really important not only to reproduce a study directly but also to extend reproduction to a larger number of situations—field studies, correlational studies, longitudinal studies.
So how do we measure racism, something that's not a single behavior but a pattern of outcomes—a whole system by which people are oppressed? The best approach is to observe the pattern of behaviors and then see what happens when we alter or control for a variable. How does the pattern change? Take policing. If we remove prejudice from the equation, racially disparate patterns persist. The same is true of poverty, education and a host of things we think predict crime. None of them are sufficient to explain patterns of racially disparate policing outcomes. That means we still have work to do. Because it's not like we don't know how to produce nonviolent and equitable policing. Just look at the suburbs. We've been doing it there for generations.
Of course, there is uncertainty. In most of this world, we are nowhere near confidence about causality. Our responsibility as scientists is to characterize these uncertainties because a wrong calculation in what drives something like racism is the difference between getting policies right and getting them wrong.
The Neuroscientist
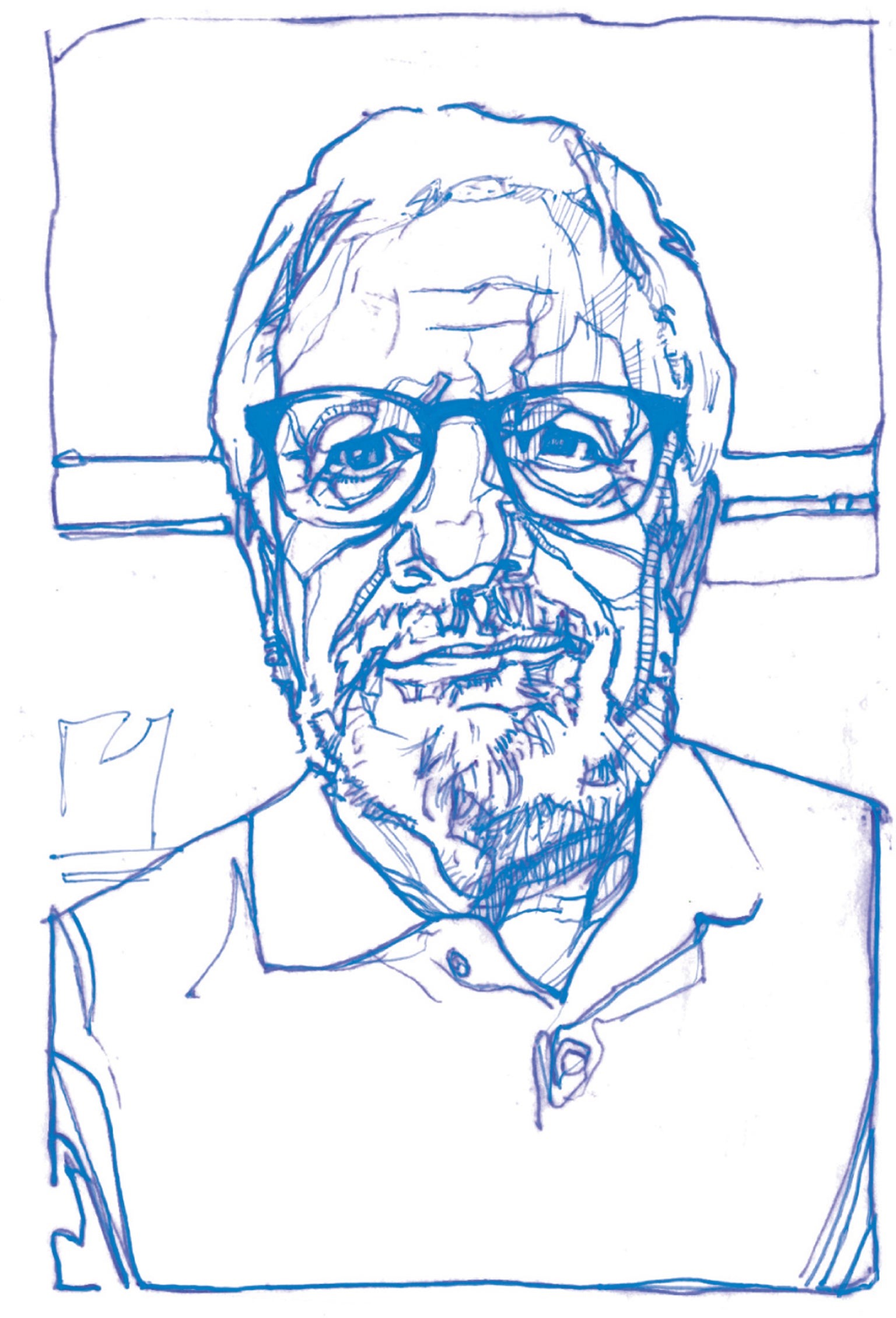
Science does not search for truth, as many might think. Rather the real purpose of science is to look for better questions. We run experiments because we are ignorant about something and want to learn more, and sometimes those experiments fail. But what we learn from our ignorance and failure opens new questions and new uncertainties. And these are better questions and better uncertainties, which lead to new experiments. And so on.
Take my field, neurobiology. For around 50 years the fundamental question for the sensory system has been: What information is being sent into the brain? For instance, what do our eyes tell our brain? Now we are seeing a reversal of that idea: the brain is actually asking questions of the sensory system. The brain may not be simply sifting through massive amounts of visual information from, say, the eye; instead it is asking the eye to seek specific information.
In science, there are invariably loose ends and little blind alleys. While you may think you have everything cleared up, there is always something new and unexpected. But there is value in uncertainty. It shouldn't create anxiety. It's an opportunity.
The Theoretical Physicist
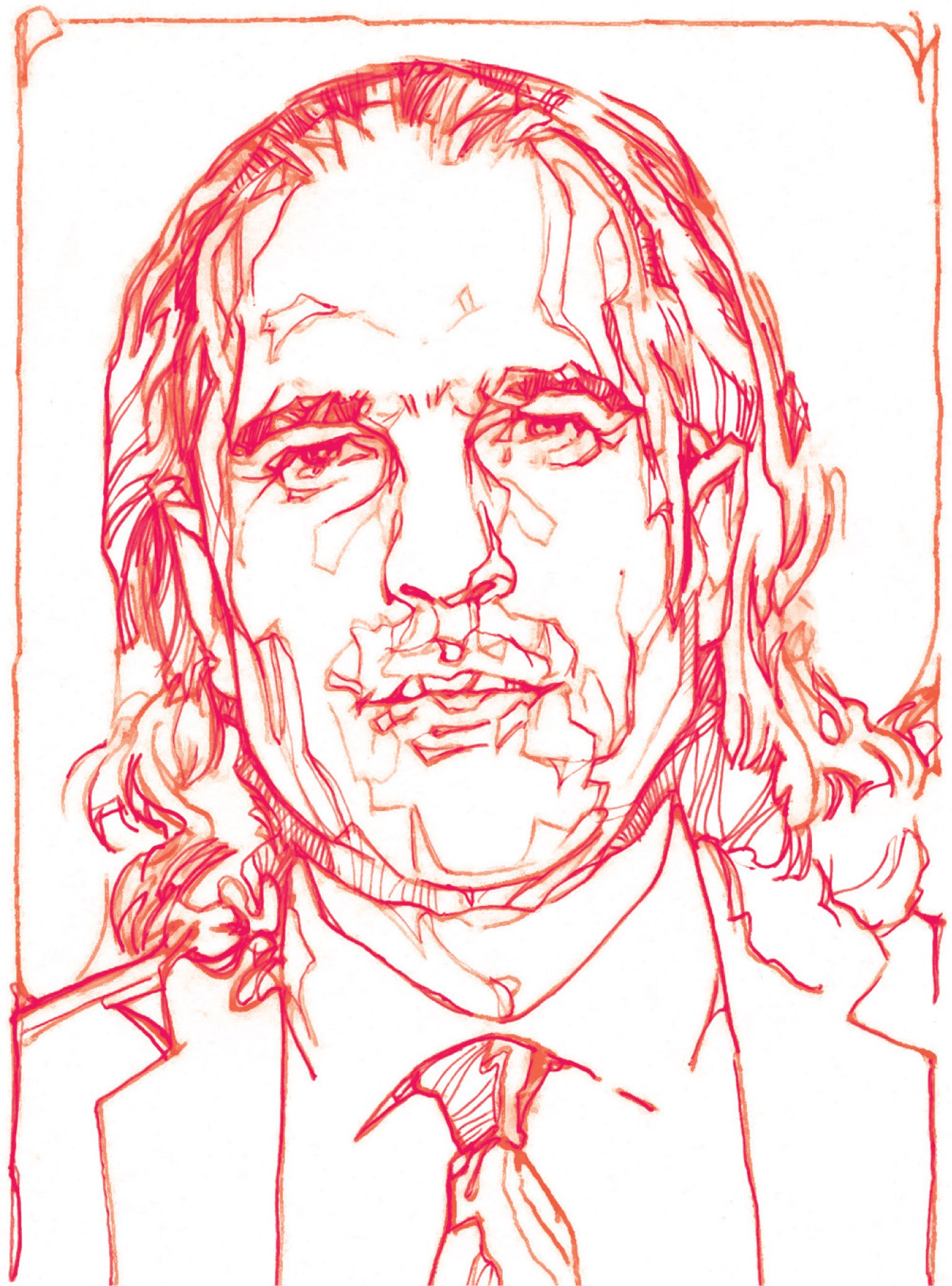
Physics is the most mature science, and physicists are obsessive on the subject of truth. There is an actual universe out there. The central miracle is that there are simple underlying laws, expressed in the precise language of mathematics, which can describe it. That said, physicists don't traffic in certainties but in degrees of confidence. We've learned our lesson: throughout history, we have again and again found out that some principle we thought was central to the ultimate description of reality isn't quite right.
To figure out how the world works, we have theories and build experiments to test them. Historically, this method works. For example, physicists predicted the existence of the Higgs boson particle in 1964, built the Large Hadron Collider (LHC) at CERN in the late 1990s and early 2000s, and found physical evidence of the Higgs in 2012. Other times we can't build the experiment—it is too massive or expensive or would be impossible with available technology. So we try thought experiments that pull from the existing infrastructure of existing mathematical laws and experimental data.
Here's one: The concept of spacetime has been accepted since the early 1900s. But to look at smaller spaces, you have to use more powerful resolution. That's why the LHC is 17 miles around—to produce the huge energies needed to probe tiny distances between particles. But at some point, something bad happens. You'll put out such an enormous amount of energy to look at such a small bit of space that you'll actually create a black hole instead. Your attempt to see what is inside makes it impossible to do so, and the notion of spacetime breaks down.
At any moment in history, we can understand some aspects of the world but not everything. When a revolutionary change brings in more of the larger picture, we have to reconfigure what we knew. The old things are still part of the truth but have to be spun around and put back into the larger picture in a new way.
This article was originally published as a series of sidebars in Scientific American Volume 321, Issue 3, (September 2019).